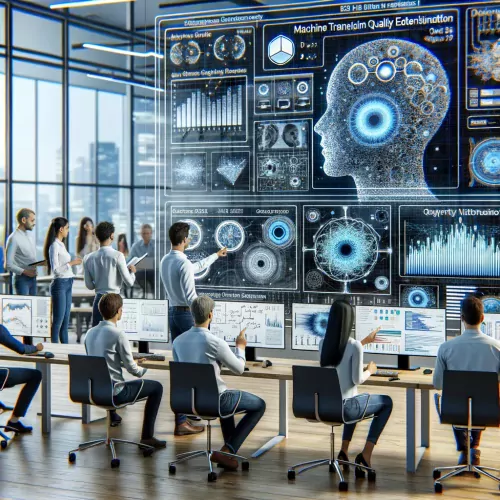
In recent years, quality estimation for machine translation has become an essential field of study, playing a pivotal role in ensuring that machine translations meet the required standards. This process, often referred to as machine translation quality estimation (MTQE), is crucial for developing reliable translation systems and enhancing their performance. The demand for accurate and reliable translations has surged with the increasing globalization and the need for cross-cultural communication. Consequently, the ability to assess the quality of translations generated by AI systems has never been more important.
Understanding Machine Translation Quality Estimation
Machine translation quality estimation (MTQE) involves predicting the quality of translations produced by machine translation systems without the need for reference translations. This differs from traditional translation quality assessment methods, which often rely on human evaluation or comparison with pre-translated texts. MTQE aims to provide real-time feedback on the reliability of machine-generated translations, facilitating improvements in both the algorithms and the translated content.
The core objective of MTQE is to develop models that can accurately predict the quality of translations. These models analyze various features of the translated text, such as fluency, adequacy, and coherence, to determine its overall quality. This process is instrumental in identifying errors and areas that require improvement, thereby enhancing the performance of AI translations.
The Evolution of Translation Quality Assessment Models
Over the years, numerous translation quality assessment models have been developed to address the challenges posed by machine translations. These models have evolved from simple rule-based systems to sophisticated AI-driven algorithms capable of understanding and evaluating complex linguistic patterns. Early models primarily focused on surface-level features, such as word order and grammar, but modern approaches leverage deep learning techniques to gain a deeper understanding of the text.
One prominent approach in this field is the development of neural network-based models, which have significantly improved the accuracy of translation quality evaluation. These models utilize large datasets to train neural networks, enabling them to learn from a vast array of linguistic nuances and patterns. By leveraging the power of AI, these models can predict translation quality with a high degree of accuracy, making them indispensable tools for machine translation systems.
The Role of AI in Translation Quality Evaluation
The advent of AI has revolutionized the field of translation quality assessment, leading to the development of more sophisticated and reliable models. AI-driven systems can process vast amounts of data, allowing them to identify patterns and make predictions with remarkable precision. This capability is particularly beneficial for machine translation quality estimation, as it enables the development of models that can assess translations in real-time.
One of the key advantages of AI-driven translation quality assessment models is their ability to adapt and learn from new data. As these models are exposed to more translations and feedback, they become increasingly adept at predicting quality and identifying errors. This continuous learning process is crucial for improving the performance of machine translation systems and ensuring that they deliver high-quality translations.
Challenges and Future Directions
Despite the significant advancements in machine translation quality estimation, several challenges remain. One of the primary challenges is the inherent complexity of human language, which makes it difficult to develop models that can accurately capture all linguistic nuances. Additionally, the quality of translations can vary significantly depending on the context and domain, necessitating the development of specialized models for different types of content.
Another challenge is the need for large and diverse datasets to train AI-driven models. High-quality datasets are essential for developing accurate and reliable translation quality assessment models, but acquiring such data can be time-consuming and expensive. Moreover, ensuring the diversity of datasets is crucial for developing models that can generalize well across different languages and contexts.
Looking ahead, the future of machine translation quality estimation lies in the continued integration of advanced AI techniques and the development of more sophisticated models. Researchers are exploring various approaches, such as transfer learning and multi-task learning, to enhance the performance of translation quality assessment models. These techniques aim to leverage knowledge from related tasks and domains, enabling models to make more accurate predictions with limited data.
In conclusion, quality estimation for machine translation is a rapidly evolving field that plays a crucial role in the development and improvement of machine translation systems. By leveraging the power of AI, researchers have made significant strides in developing accurate and reliable translation quality assessment models. These advancements have paved the way for more sophisticated and effective machine translation systems, capable of delivering high-quality translations across various languages and domains. As the field continues to evolve, the integration of advanced AI techniques and the development of specialized models will be key to overcoming the remaining challenges and ensuring the continued success of AI translations.